- Data Drift: This can happen if the model is not exposed to new data or if the data it is exposed to is not representative of the real world.
- Concept Drift:Â This can happen if there are changes in the way data is collected or if there are new factors influencing the data.
- Adversarial Attacks: Malicious actors can intentionally manipulate data to intentionally harm or mislead an AI model.Â
- Overfitting: An AI model may become too closely tied to the training data, making it unable to generalize well to new data.
- Algorithmic Errors: Errors in the AI algorithm itself can also lead to performance degradation over time. These errors can be introduced during the development process or they may arise from unintended interactions with the data.
- Continuous Monitoring and Evaluation: This involves tracking metrics like accuracy, precision, and recall.
- Adversarial Robustness Training: Train AI models to be resilient to adversarial attacks by exposing them to carefully crafted malicious inputs.
Yes, AI models can get worse over time due to various factors
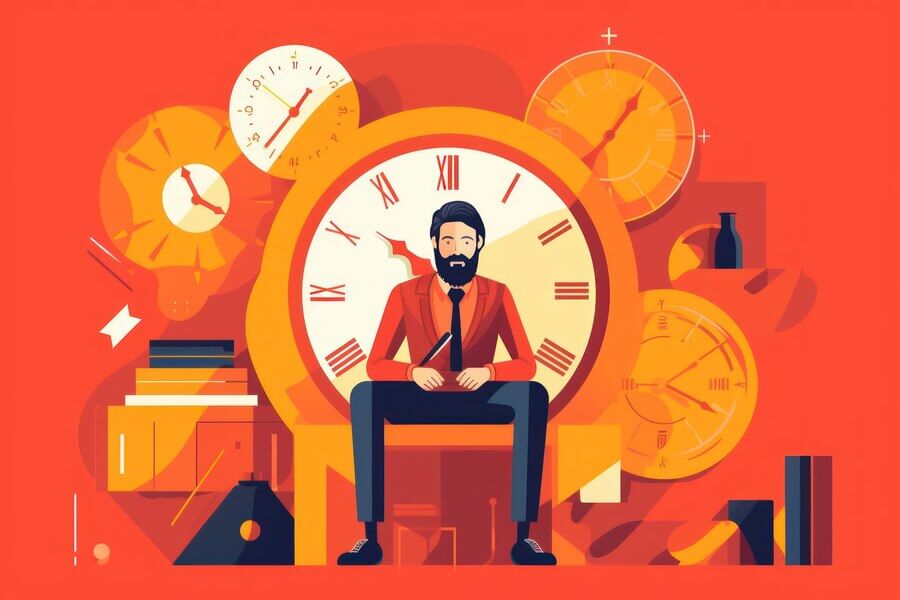
Thank you for your sharing. I am worried that I lack creative ideas. It is your article that makes me full of hope. Thank you. But, I have a question, can you help me?